Understanding a Data Mesh
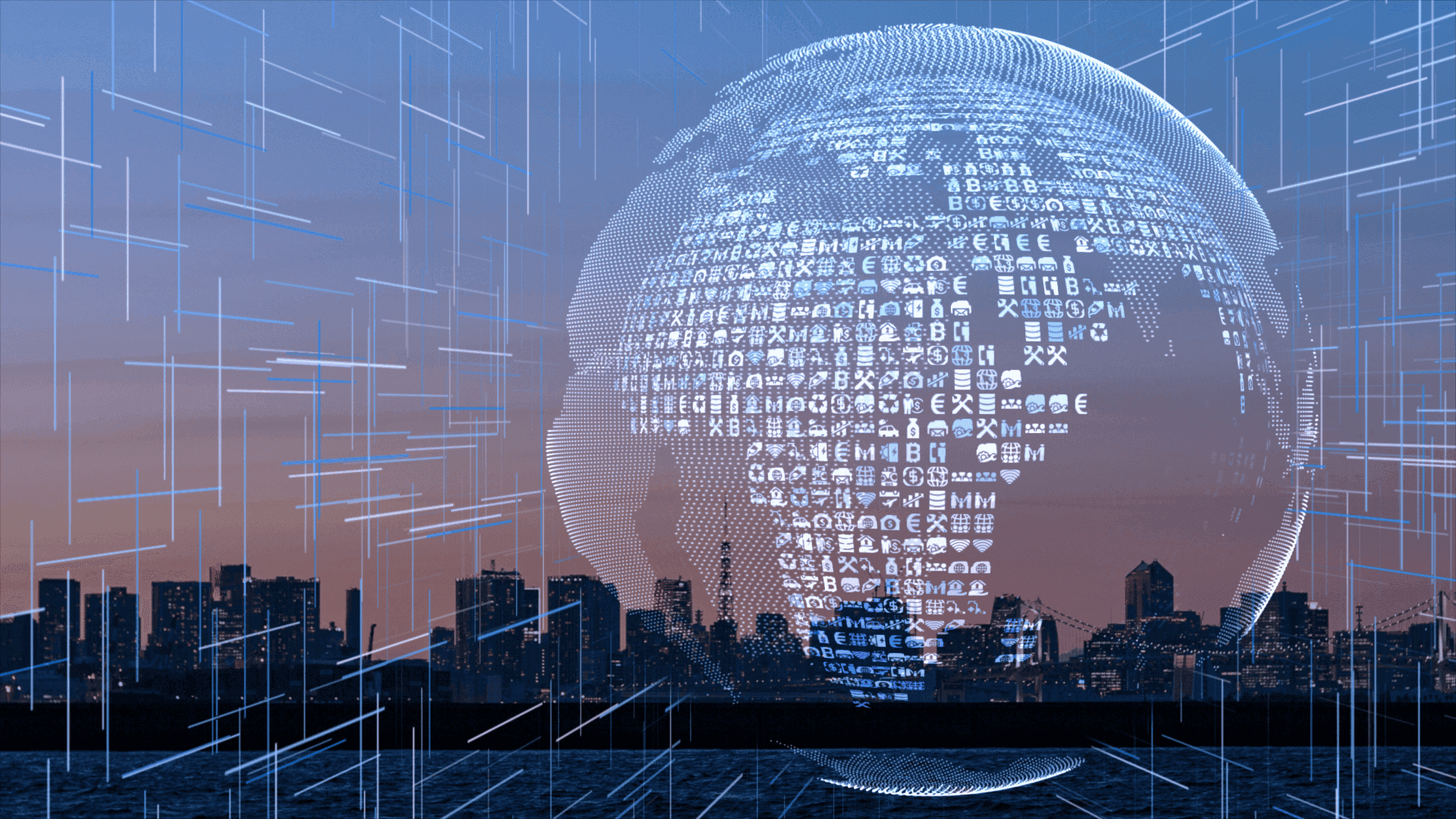
Self-Service Business Intelligence is in the forefront in most of the organization and with this, the importance of data increases. Companies are defining themselves as data-first organizations but do all of them provide an open and scalable data architecture? With demands to integrate more and more data sources through a single ETL pipeline and dealing with an endless stream of ad hoc queries, every data team wishes there is a simpler approach to meet these needs.
This is where a Data Mesh comes in. To being with, we need to understand that Data Mesh is a process and NOT a technology. A frequent problem each organization faces is the continuous disconnect and discontent between the IT and Business Teams. With IT teams complaining of not getting the complete requirements and the Business team complaining of having to deal with the IT team for every requirement, this Data Mesh leaves it now to the business teams to manage and maintain their own setup. In other words, the Data Mesh allows for “Decentralized Data Ownership and Curation.”
With a purpose to provide data owners with more autonomy and flexibility, it provides data teams with an architectural paradigm that decentralizes data ownership and management across multiple domains and business units. The whole aim is to distribute the data infrastructure thereby enabling domain specific teams to manage their own specific data pipelines, schemas, and governance.
There are 4 major principles governing a Data Mesh:
- Domain Centricity – Every domain manages their own data pipelines, infrastructure, and schemas independently.
- Domain-Oriented Decentralized Data Ownership – Data Management and ownership is split across teams and domains allowing for greater accountability and domain expertise.
- Product Mindset – The approach is to treat Data as a Product and thus promoting self-service data platforms for domain-based teams with clear ownership, consumption APIs and quality standards.
- Federated Data – Increased emphasis on Data Sharing and Collaboration across org boundaries. Coupled with a Self-Service functionality, this supports many business roles.
A simple example of a Data Mesh is when in a large MNC, each business unit (like Sales, marketing, finance etc.) has their own independent Data Team. Through this Data Mesh, each team can manage their own Data Pipelines, schemas, and analytics that is specific to their team and domain. Standardized APIs and Data Catalogues could be shared across them for cross-domain insights.
Building a Data Mesh is enabled through the presence of technologies like Snowflake Data Cloud wherein organizations can create multiple DBs via different teams but still retain the ability to share across them for other use cases. The core principle driving this is that every unit treats its data as a product and so they acknowledge that they need to provide a data service to the other teams through the availability of APIs to pull their data when needed.
Here is how we @ Tarento as an implementation partner for one of the Global Investment Firms, helped our client to take advantage of Data-As-A-Product via a Data Mesh Implementation.
What did this Data Mesh help our partner achieve?
The main benefit was to speed up the time to deliver analytics and business intelligence. The centralized IT team took months to integrate new data sets that were needed for analytics in addition to having data quality issues due to limited Domain understanding. This is what the Data Mesh exactly helps to alleviate. It increased the speed of delivery and the ability to collaborate with other data teams within the organization.
Like we face in the real world, benefits do come with their associated challenges.
- With decentralized ownership, it runs a risk of data silos appearing across domains, leading to potential inconsistencies and difficulties in achieving a unified view of enterprise data.
- Maintaining global data governance and adherence to standards becomes more complex in a distributed architecture.
- Successful implementation requires significant readiness and a cultural shift towards domain-driven data ownership.
To summarize, a Data Mesh is a Company Focussed Analytics Architecture wherein each business unit within the organization has its own analytics infrastructure and data team to maintain it but all guides by company-wide governance policies. While it empowers business domains to develop tailored data products, it also has its own set of challenges. As it matures, we would see development of more robust tools and frameworks to support federated data governance, cross-domain data lineage tracking, and automated data product discovery and management. Add to this the ever-increasing usage of AI and ML and it would allow for automating data quality checks, metadata management and data product recommendations across domains.
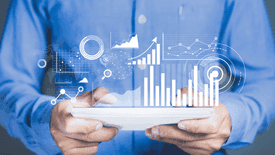